Industrial Internet: Big Data and Analytics Driving Big Outcomes
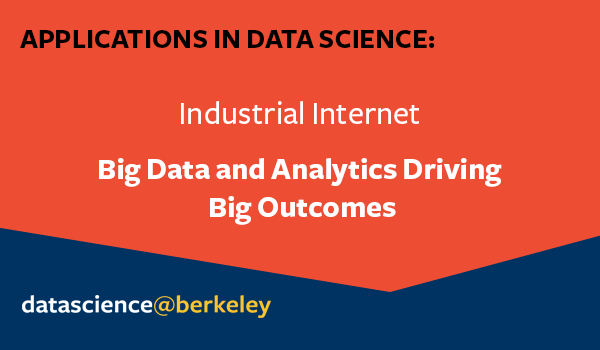
The torrent of data generated from machines, networks, devices, and data centers in industry verticals provide challenges and opportunities. The challenge is to make this machine data meaningful and actionable to deliver on opportunities around operational efficiencies. On March 15, Beena Ammanath, Vice President of Data Science at General Electric, joined datascience@berkeley to present “Industrial Internet: Big Data and Analytics Driving Big Outcomes.”
In the webinar, Ammanath shared real-world case studies demonstrating tangible operational benefits by tightly integrating machines, networked sensors, industrial-strength data, and software to enable intelligent insights and affect measurable outcomes. Here, we provide a recap of her presentation and key takeaways about how the Industrial Internet is making the most of big data to drive big outcomes.
Dynamics of the Industrial Internet
Referencing the significant impact of the industrial revolution of the 1990s, Ammanath says there is a new and similar revolution emerging—sometimes referred to as the Next Industrial Revolution, or Industry 4.0. It’s being driven by the Industrial Internet, which is creating big results in the process: “The outcomes we drive leveraging big data or AI are big because of the sheer impact it will have on all of us. Pretty much every single day industrial companies impact your life.”
With the emergence of the Industrial Internet, industrial companies are realizing that software and analytics must now move to the core of their strategies—alongside their traditional industrial equipment and related services strategies. Because of these dynamics, data science is becoming a competitive differentiator in asset-heavy companies.
Just as the Consumer Internet emerged two decades ago—when one 1 billion people began connecting in a variety of ways—Ammanath believes the same thing is happening in the industrial world as billions of machines will be increasingly connected in coming years. This intelligent network of industrial machines working together is what she is referring to as the Industrial Internet.
Since analytics failures in the Industrial Internet can have more severe consequences than the Consumer Internet, it has three components that are both essential and somewhat unique: reliability, security and durability. In addition to ensuring that systems will function as expected, it’s also critical that data breaches are prevented and that software lifecycles align with those of the machines that contain them.
Industrial data gathering and management
In several examples, Ammanath demonstrated how data gathering and analysis help to optimize industrial assets and operations and reduce unplanned downtime. Tiny sensors play a major role—with thousands attached to big machines, enabling the capture of real-time data that is then wirelessly streamed to the cloud where it is engineered and analyzed by powerful software. The software then proactively generates alerts so that the necessary preventive steps can be taken to prevent machine failures and optimize efficiencies.
When it comes to data management, Ammanath says the approach that industrial companies have traditionally used doesn’t work anymore. It’s too slow, too expensive, and too rigid for the use cases that need to be addressed today. As a result, companies are now building data lakes to serve as enterprise-wide data management platforms for analyzing disparate sources of data in native formats. Once data is placed into the lake, it’s available for analysis by everyone in the organization. However, additional resources are needed for data lakes to be effective—which is why new roles are evolving to support their consumption.
Primarily, data lakes try to reduce silos and make valuable information more accessible. The big issue with this approach is that it makes certain assumptions—that all users:
- recognize and understand the contextual bias of how the data is captured;
- know how to merge and reconcile different data sources without any prior knowledge; and
- understand the incomplete nature of the data set, regardless of structure.
However, most business users lack this level of sophistication. That’s why companies have teams of data engineers—sometimes referred to as data janitors—who focus on building out a consumption layer for the end-user, whoever that may be. This allows any end-user to access the underlying data quickly and seamlessly with the right level of context. Ammanath says this is the key to succeeding in data science in an industrial world: being able to put context around any machine data to make it usable by any end-user.
Industrial outcomes
Although analytics are essential to creating outcomes, using the former doesn’t directly result in the latter. Instead, Ammanath says that depositing clean data in a big data environment only results in connections and correlations: “What you get at the end of the day is a formula. An output that still needs a human brain to interpret the correlation, to understand it, to contextualize it, and wrap it in a form that can then be easily consumed by either humans or machines to drive real outcomes.”
She says it’s something her company has been working on: “We are aware of that. We have been building and consuming physics-based and statistics-based algorithms in the industrial companies. But with the newer technology we are able to fuse machine learning with the traditional data science approach to drive bigger outcomes leveraging our in-house domain knowledge, marrying it with the newer technologies.”
Artificial intelligence in the factories
Ammanath defined three levels of artificial intelligence (AI): narrow, general, and super. “Artificial narrow intelligence is a form of AI that that is equivalent or better than human intelligence at solving a very specific, narrow problem. Artificial general intelligence is intelligence that matches a human level of intelligence to solve any kind of problem that a human can solve intellectually. Artificial super intelligence is smarter than the best human brains in practically every field.”
She notes that when AI is referenced, it’s mostly related to artificial narrow intelligence, both in the consumer space and the industrial space. For example, being able to mine through petabytes of data to predict when a power grid might fail allows a company to proactively send field engineers to fix the issue before a power outage occurs. However, Ammanath says that’s the extent of its functionality at this point, and it cannot independently extend itself to make the repair.
Yet.
Although the software or hardware needed to build artificial general intelligence or super intelligence isn’t yet available, she sees hope in the future: “The human brain is incredibly complex. Building the software and analytics techniques to mimic our brain is unbelievably challenging. What can be really easy and simple for the human brain is unbelievably difficult to program and compute. But that’s exactly the challenge that you all have in front of you, to figure that out.”
She says the topic is something she’s very excited about: “Because when we do start down the path to super intelligence, the impact to all humans is something that we can only imagine. Super intelligence will have all the intelligence of the smartest human beings combined. Can you imagine what our world would look like with artificial super intelligence?”
Noting that the Industrial Internet could have a $15 trillion impact on the global GDP over the next 15 years, Ammanath closed with this prediction: “The Industrial Internet is going to have some of the biggest impact transforming economies, saving lives, reducing power consumption, and changing the way we live.”