DataBeat 2014: Splunk Slices Data for Domino’s Pizza
The following post explores just one of the many talks at DataBeat 2014. To find out more about DataBeat 2014, check out our recap here.
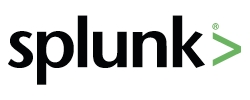
If you’re in the marketing or analytics space, you’re no doubt familiar with traditional metrics like time on site, page views, and revenue returns — numbers that are actively collected and later made actionable by marketers and managers.
But when was the last time you analyzed machine data? This data, also known as “data exhaust,” is the focal point of Splunk’s business. It includes “byproduct” data from things like GPS, RFID, hypervisor, web servers, e-mail, messaging, clickstreams, mobile, telephony, databases, and more — data that is created simply by the act of running a machine.
This data derives from outside traditional “data science” parameters. Think, for example, about the information collected from transportation (the operation of trains, planes, and elevators all creates massive data sets with little organization) and health care devices (hospital health monitoring machines and wearable tech like Fitbits all lead to unstructured data “exhaust”). In fact, machine data is a key byproduct of the Internet of Things. As more and more of our daily activities are monitored or occur via connected devices, more data will be collected without a goal in mind.
Machine data is one of the fastest growing and most complex and challenging aspects of big data, and one which certainly meets the criteria for the four V’s of big data: volume, velocity, variety, andvariability. Splunk wants to make this largely unstructured data accessible, usable, and valuable for everyone.
What are their customers doing with machine data? As it turns out, they’re employing these data sets in everything from app management and development to IT operations, security and compliance practices, and even traditional business analytics. Let’s take a look at some of Splunk’s clients:
Domino’s Pizza
Domino’s Pizza has long used machine data to keep their websites running smoothly. During big events like the Super Bowl or the World Series, the company needs to make sure their website stays up and running so it can serve their huge (hungry!) clientele. However, there’s a problem — machine data is distributed across many different systems.
The company started using machine data for troubleshooting, and now use it for continuously and actively monitoring systems. Using Splunk’s technology, Domino’s can collect real time revenue insights, online statistics from over 10,000 stores. This allows them to target campaigns and tweak coupons in real time, leading to device and promotion improvements that positively impact their bottom line.
New York Air Brake – Dynamic Systems Division
New York Air Brakes uses byproduct machine data for a wide variety of things, including remote freight train monitoring, energy efficiency calculations, and driving strategy recommendations. Guido Schroeder, Splunk senior vice president of products, estimates that the company has saved over $1 billion yearly simply by crunching these numbers and adjusting based on the results. How?
In one example, 5,000 trains were equipped with sensors that passively monitor things like fuel consumption and forces exerted on moving trains. By making adjustments accorded to issues found in the data, the trains saw improvements between 8 and 12 percent. When you apply those results to the $10 billion spent each year in fuel expenditures, it’s easy to see how the industry saved $1 billion a year simply by listening to their byproduct machine data.
These trains have become incredibly energy efficient — nothing like your car. In fact, one ton of a freight train can be moved 500 miles on just one gallon of fuel. Now, with efficiency concerns out of the way, engineers can return to the same machine data sets and focus on improving safety of the trains. Algorithms can be put in place that alert drivers to safety issues, thereby improving the safety records of trains. In a $70 billion industry, data collected “on the go” can make all of the difference in operating efficiency, safety, and revenue returns.
Want more from DataBeat 2014? Check out our other posts: